This post provides an overview of what has recently changed in the digital pathology and image analysis offer on the market
Artificial intelligence (AI) has already entered various fields of medicine with great success. With a growing acceptance of AI in the pathologists’ community and application of deep learning methods to solve pathology problems, tech startup companies are popping up like mushrooms and are starting to explore the digital pathology niche. As the dimensionality of pathology problems is very high compared to other medical disciplines, the potential for applications of trainable deep learning models is expansive. For example:
- Detection of sclerotic glomeruli in the kidney
- Detection of H.pylori gastritis
- Liver steatosis quantification
- Liver fibrosis quantification
- Region-based PDL-1 scoring
- IHC positive cell quantification
- Special stain quantification
- Inflammatory myopathy quantification
- You name it!
These and many other problems can be addressed with deep learning models. Deep learning is a powerful technology, and often for the users with no image analysis background (e.g. pathologists), it is currently easier to use artificial intelligence, than the classical image analysis methods. The interface to annotate structures of interest and train the deep learning model to detect them independently later (correcting on the way, when reinforcement machine learning is incorporated into the software) is a lot easier and user-friendly than manually defining and adjusting the parameters of the structures you are trying to identify. With deep learning models, you don’t need to endlessly adjust and tweak the thresholds of your parameters to fit your images. You simply give appropriate examples and the model learns. The more different examples you give, the better it should learn.
As a response to the wider AI acceptance there has been a paradigm shift in the digital pathology industry:
- Image analysis companies are abandoning the classical hand-crafted image analysis algorithms and pushing strongly towards deep learning, either as customized solutions integrated into the users’ existing computerized systems thanks to the open application programming interface (API) or integrating the deep learning modules into new or existing software packages.
- More and more companies are providing software as a service (Saas) instead of traditional computer installed software. To enable users who do not dispose of enough computational power on their premises to run deep learning models efficiently, companies are now also offering cloud computing capabilities and cloud-based user interfaces.
- Companies, instead of designing software that makes the decisions for the users, now develop decision support systems, where pathologists and scientists have the final word and can accept or reject the results suggested by the software.
- Digital pathology and image analysis software is becoming more and more user-friendly.
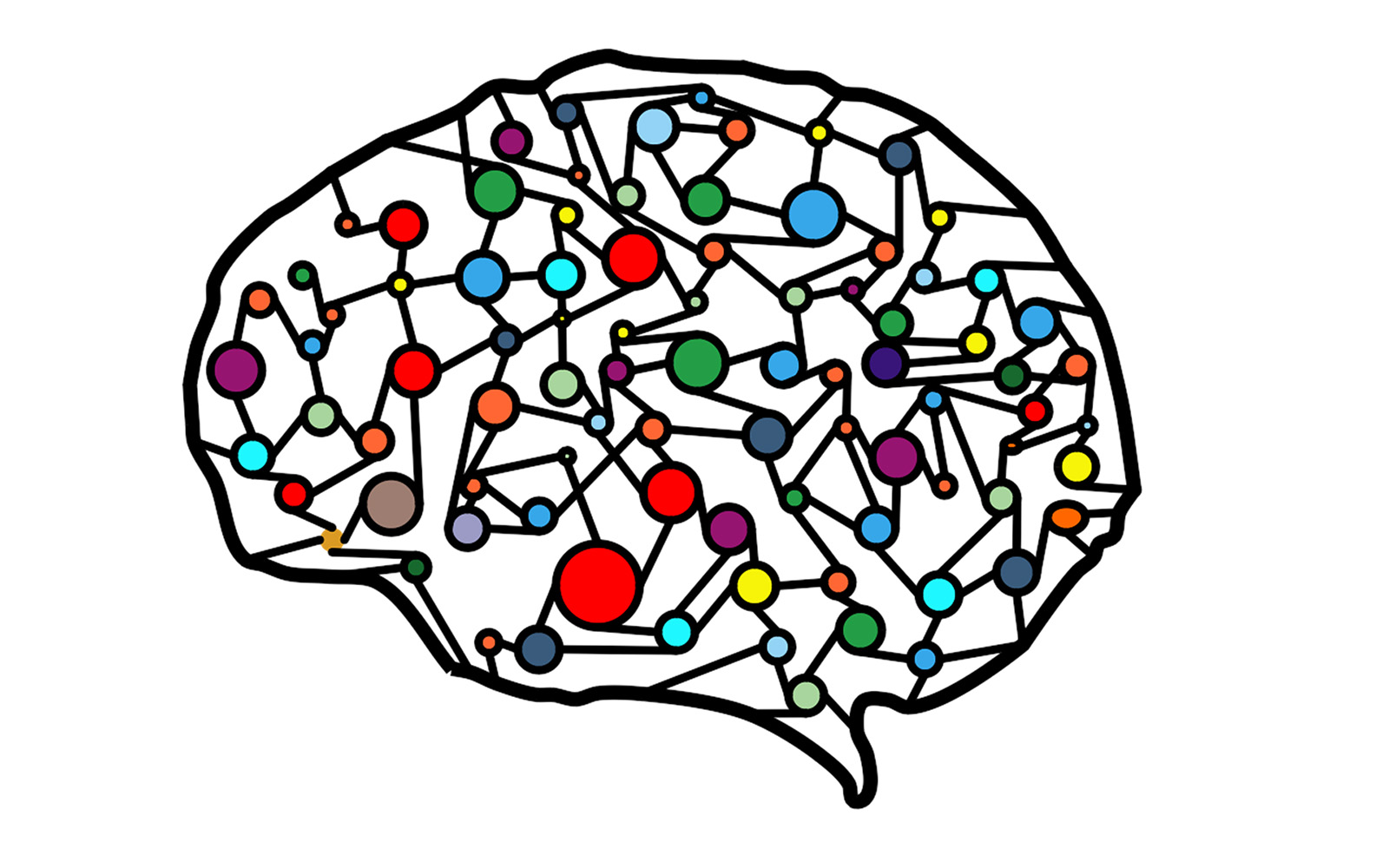
Push towards deep learning
Let’s face it, classic image analysis software, be it open source or commercially available, is not very user-friendly. You must open one drop-down menu after another, which are embedded in each other, in order to perform a single action. The learning curve is steep, it takes time and you forget it easily once you stop using the software regularly. This is the cost of providing all the functionalities of the software to users who are not programmers. At some point, the graphic user interface just becomes too complicated. On top of that manual selection of features on which image analysis should be based is limiting and not generalizable in heterogeneous data sets. As deep learning and artificial neural networks proved to be performing well in computer vision tasks, this seemed to be the natural evolution of image analysis programs. Many companies (like Aiforia) are creating systems solely relying on deep learning whereas the “veterans” of the digital pathology market (like Visiopharm and Indica labs) added the artificial intelligence modules to stay competitive.
The artificial intelligence-based image analysis systems let the user give the system examples without the need to precisely define the detection criteria. Based on the given examples (aka. labeled data, annotations) the system learns to recognize structures on unseen images. Later the performance can be reviewed by the user and improved by giving more examples and/or correcting the misclassified structures. Some programs like the Studio by DeePathology are even able to guide the example generation in such a way that the user knows which structures are most ambiguous for the model and can specifically increase their number in the training set. This significantly lowers the bar for adoption even for the more skeptical users. The incorporation of image analysis into the workflow is now much easier and due to the open API chosen by many of the newer market players the user-generated solutions can now be integrated into other computerized systems, like the lab information management systems (LIMS).
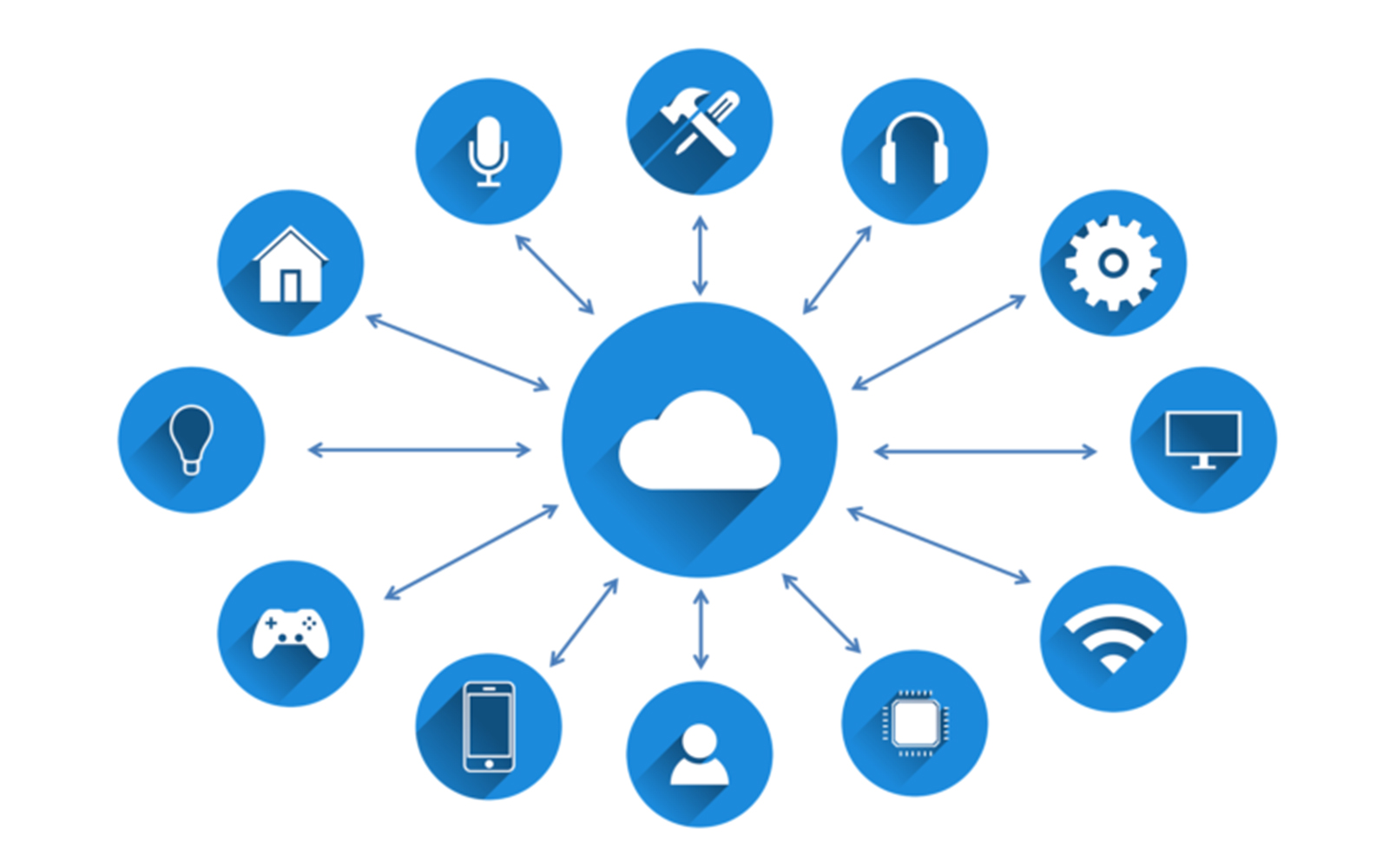
Moving into the cloud
Deep learning, as powerful and user-friendly as it can be, is not free of its challenges. One challenge is the need for more powerful computers to process the images. An ordinary laptop will not suffice anymore to generate results in a reasonable time. The computers used for training deep learning models should be equipped with graphics processing units (GPUs) which increase their speed but also increase the cost significantly. Having to change your equipment to start using deep learning-based image analysis is a big hurdle. In response to that problem, companies started moving their services into the cloud. To access the user interface and upload the images you just need to log in via your internet browser. You provide the labels there and all of the computations take place on remote servers via the Internet, providing the user with rapid results. No need to change any equipment and pay the high maintenance cost of the infrastructure. You get access to the computational power you need and some companies even offer a “pay as you use” pricing model, which charges you for the computational resources that you actually use (after paying an initial access fee which is significantly lower than the traditional software license).
Another advantage of cloud-based systems is, that especially in large organizations, there is no need to integrate another software into the IT infrastructure. Rather the system is already integrated, through the internet browser. It is sufficient to only validate the recommended browser (which in many cases is Google Chrome, usually validated for the company’s use anyway) and we are ready to go and design our own deep learning models.
Disadvantages of cloud-based systems include the many unanswered questions about the security of remote access and the firewall between the different system users. It may very well be possible or even probable, that competitors are using the same cloud-based system. Where are their data stored and how is it secured, such that no one other than the data owner sees it? Is there a possibility of a physical audit to the company providing cloud-based solutions? How would the audit be conducted if the servers with data were placed in different locations, sometimes even on different continents? Depending on the project some or all these questions need to be addressed before starting a collaboration so that both parties – the users and the providers – are confident moving forward.
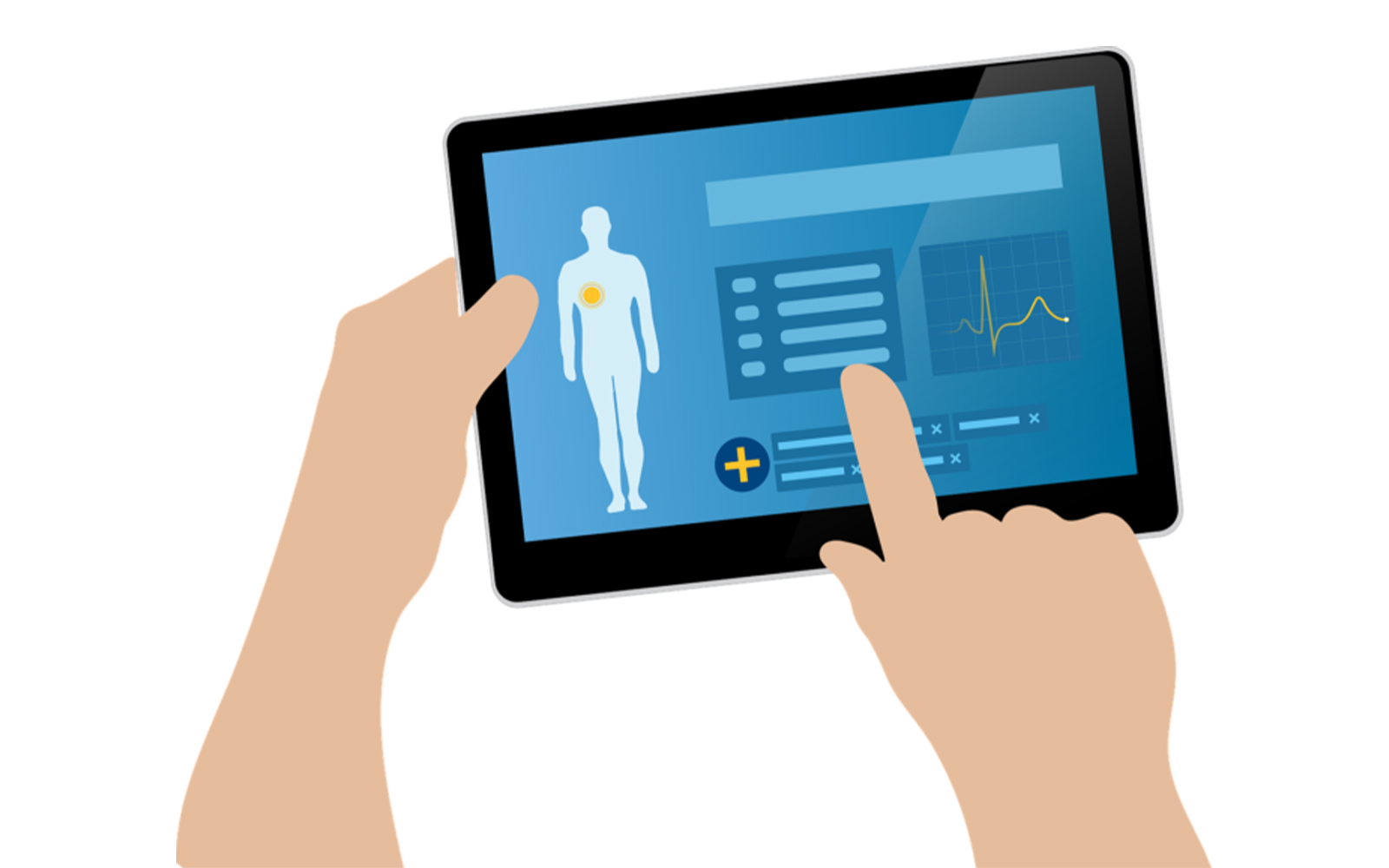
Pathologist’s decision support systems
The recent acceptance of deep learning as an integral part of digital pathology is associated with the shift from standalone solutions towards pathology decision support systems.
As pathologists, we are responsible for every case we diagnose and every slide in the study we evaluate. Letting the computer analyze something for us and not being able to influence it frightens us, and rightly so. There are very few algorithms or digital solutions for pathology, which are mature enough not to need pathologist’s supervision. Lack of AI FDA approved pathology tools reflects that. The digital pathology companies have slowly begun to understand this and adapt to the needs of the pathologists. The new trend is to show the pathologists the results of the computer software and let them decide whether it is good enough or not. This is much more adapted to the current pathologist workflow, where they must review every slide anyway. Highlighting suspicious areas can significantly accelerate the review process, adding great value.
However, the acceleration of the process cannot compromise the quality of the sample assessment in any way. Faster also needs to mean better, not worse. Only in this case will any technology advancement be justifiable. Regardless of the tools provided, the pathologist is still ultimately responsible for the sign-out, and they need to stay as professional and thorough as they were when they were working without computer assistance. They need to have the same level of confidence in the “accelerated” sign out as they have in the classical, manual one. They are still responsible for finding the hidden corner of the slide containing a micro-metastasis, even if it was not detected by the algorithm. This remains the pathologist’s responsibility, and only if it stays that way, will pathologists get on board and become more open to experimenting with new methods and technologies targeting their efficiency.
As in the Pap smear review example, where the cytotechnologist screens the samples and the suspicious cases go back to the pathologist, a percentage of the negative cases also needs to be checked for quality assurance. In the Pap smear case 10% of the negative cases should be evaluated by the pathologist. How much will it be when instead of a cytotechnologist AI will be our assistant? Nobody knows yet as there are no AI-based pathology solutions that have undergone the scrutinous review by the FDA. We will have to figure it out. Will it be 10%, 50% or will we need to review all negative cases? This probably depends on the robustness of the AI model we will be using. Would a necessity to review 100% of negative cases still accelerate our work? This probably depends on how much faster we will be evaluating the positive cases with AI assistance.
Currently, toxicopathologists and scientists responsible for drug development are looking into unsupervised deep learning methods to differentiate normal tissues from abnormal tissues and highlight the “abnormalities”. The detected abnormalities may be anything the system did not learn from the normal control group, but the decisions about their relevance are left to the pathologist. As toxicopathological studies contain hundreds (and sometimes thousands) of slides, this may have the potential to significantly accelerate the drug development process.
Will AI deliver on this promise? Time, reality and regulatory authorities will verify. It is high time to start working closely with the authorities regulating the drug development process. It is possible to use digital pathology and image analysis for Good Laboratory Practice compliant studies, but someone needs to take the first step and perform extensive validation. The first one who does that will pave the way for others and will immensely advance toxicologic pathology. It will be a great effort, but so was the Philipps IntelLiSite validation, where the company recognized the enormous value of this process and successfully paved the way for others.
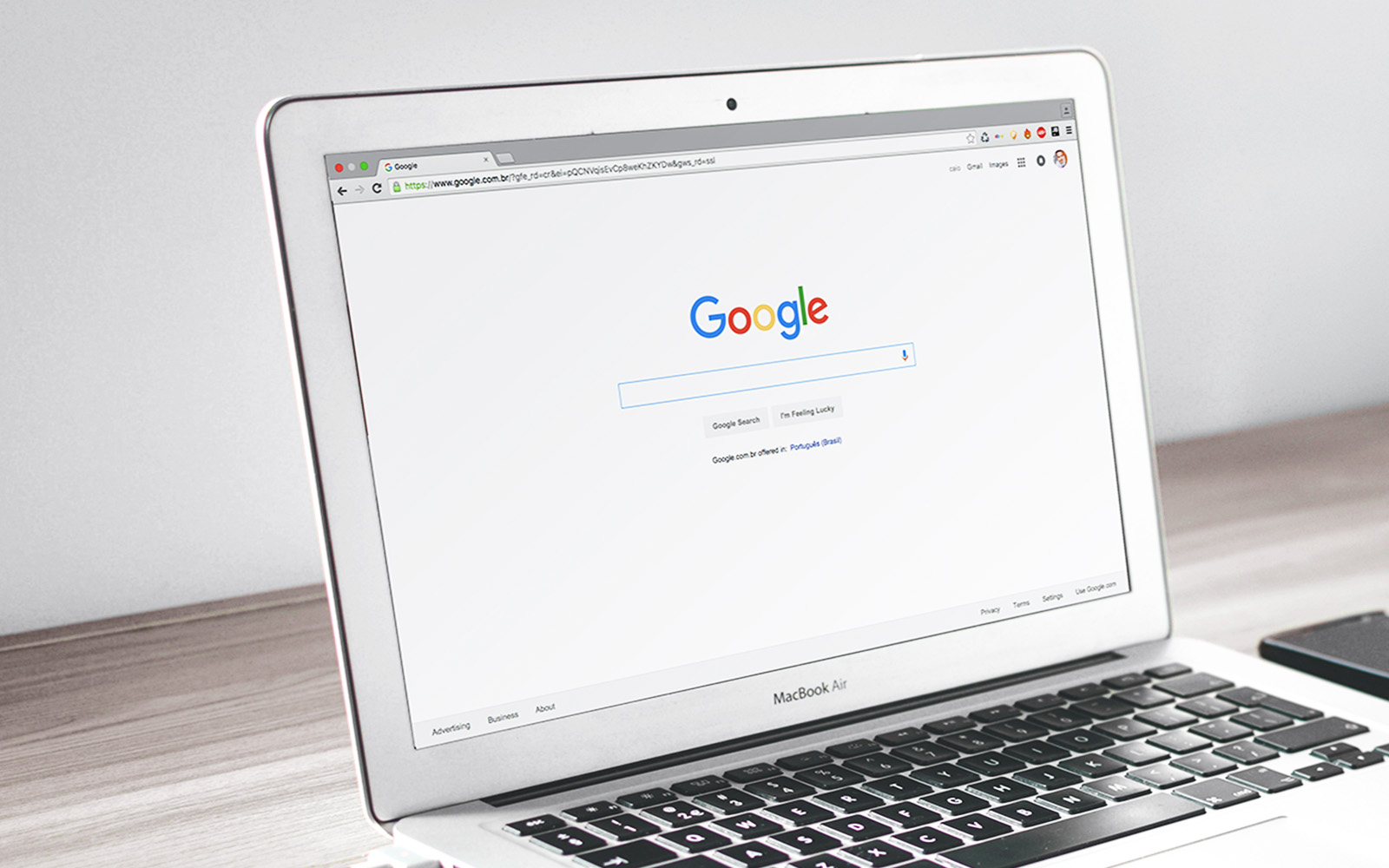
User-friendly
Another paradigm shift (occurring somewhat late, given that pathologists are supposed to be end-users of the digital pathology software) is the shift towards better usability. The software is becoming more user-friendly.
For a long time, digital pathology software looked like it was designed by the software programmers for other programmers, maybe less advanced ones, but programmers. This is still visible in some software packages where instead of clicking “yes” or “no” you still must type the truth value “1” or “0”.
Really, truth value? As an answer to a yes or no question? This is not pathologist friendly!
The user interface is changing both for IA software (especially the newer players on the market like Aiforia have it as a priority) as well as for slide viewers and slide management systems. The workflows for clinical and diagnostic pathology and for toxicologic pathology supporting the preclinical phase of drug development differ significantly. For a long time, toxicopathologists had to work with slide viewers optimized for diagnostic pathology, which made them angry and discouraged them from digital pathology. However, they didn’t really have a choice as there was nothing else available on the market. Now there are companies like Deciphex focusing only on toxicopathologists and their slide viewer Patholytix preclinical is optimized for the preclinical pathology workflow.
Additionally, image analysis solution providers recognized the plethora of pathology problems that can be addressed with AI, but rather than designing hard-coded solutions and later selling them to pathologists who may have completely different problems, they made the deep learning methods accessible to pathologists as a customizable tool for solving their particular problems. Pathologists and researchers can now train and correct the models on demand and once a robust algorithm is built and validated, it could be integrated into their daily workflow, like the H. Pylori Decision Support System designed by DeePathology with their software The Studio.
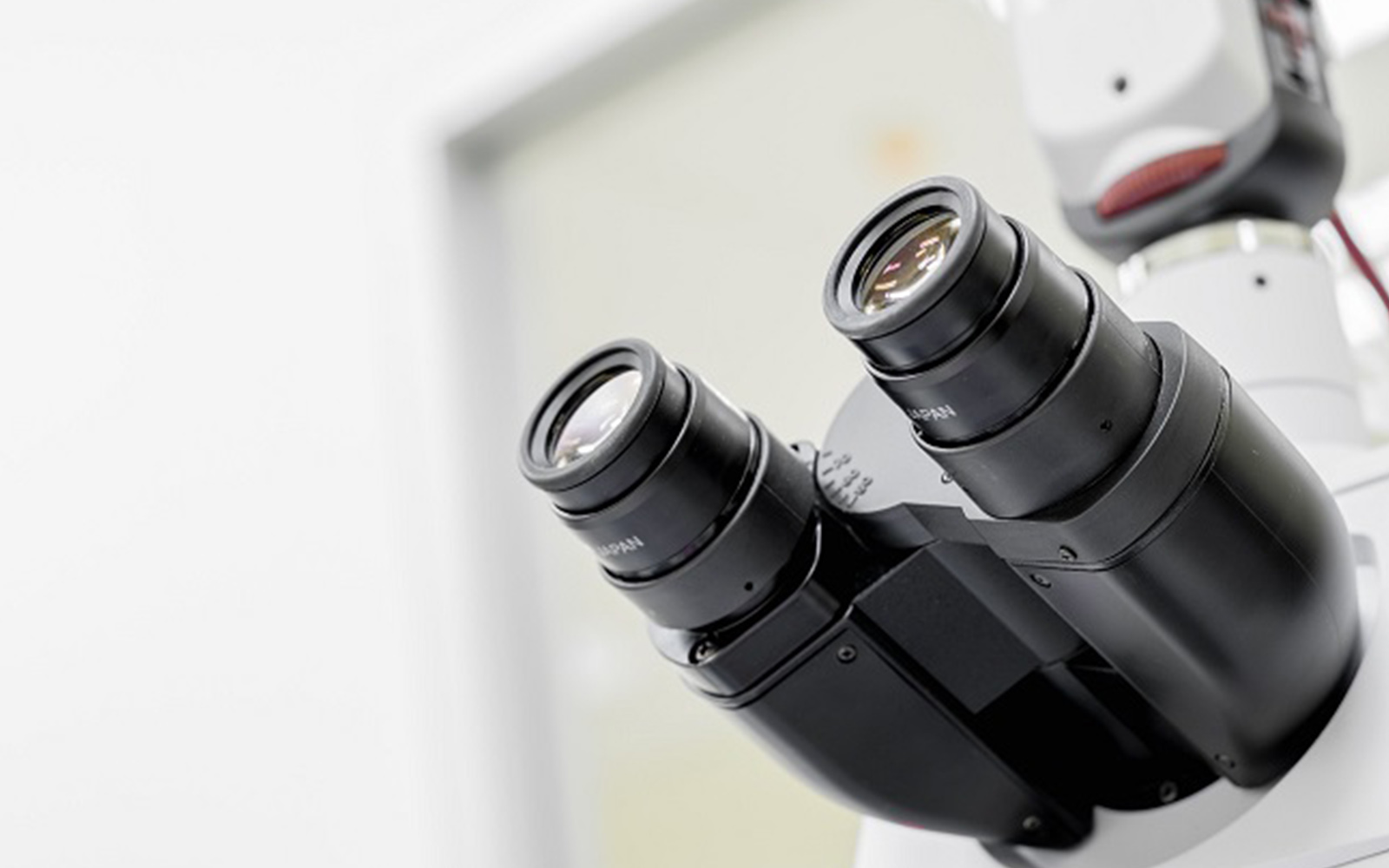
Digital pathology inside of the microscope
The digital pathology providers even started providing solutions (hardware and software) for those who want to keep working with the microscope. The company Augmentiqs provides an alternative, flat camera unit (aka augmented reality module) which can be attached to your own microscope between the objectives and the eyepiece to enable working with digital and analog pathology at the same time. The image from the microscope is transmitted live to the computer where not only can it be shared live with collaborators, but also digital image analysis algorithms and deep learning models can be applied. This gives it a great advantage over a c-mounted microscope camera for capturing static images, where the live sharing experience is either not possible or suboptimal. No matter how far the digitalization of pathology progresses, microscopes and light microscopy are the basis of current pathologic evaluation and are here to stay. Maybe in the future, they will be available just as back-up equipment, but in many regions of the world, from the rural mid-west of the United States to Malawi in Africa as the only tool of pathological evaluation. The fact that microscopes will remain in the pathology world along with the augmented reality units contributes to greater adoption of digital pathology even among the more skeptical pathologists, as well as empowers the institutions that cannot afford to go fully digital in their laboratories.
Summary
In the era of personalized medicine, where doctors and pharmaceutical companies strive to provide individualized therapy for each patient based on their biomarker profiles, also digital pathology companies realized that the “one size fits all” approach is not the best model for pathologists. Every institution has its own specific workflow and its own challenges, and it is not possible to develop one product for everybody. The solutions designed for primary diagnosis in a very busy hospital usually don’t fit the flexibility and workflow requirements of research or drug development support team.
To better address the customers’ needs paradigms in the digital pathology industry needed to shift:
- Image analysis companies replaced the classical hand-crafted image algorithms with trainable deep learning methods.
- More and more companies are providing software as a service (Saas) instead of traditional computer installed software.
- To enable users who do not possess enough computational power on their premises to run deep learning models efficiently, companies now offer cloud computing capabilities and cloud-based user interfaces.
- Companies, instead of designing software that makes the decisions for the users, now develop decision support systems, where pathologists and scientists have the final word and can accept or reject the results suggested by the software.
- Digital pathology and image analysis software has become more and more user-friendly.
These together with paying close attention to the users’ needs and working closely with them to improve existing products is yielding more and more personalized digital pathology, where the state of art computer vision and image analysis methods can be used flexibly and applied to a variety of pathology and scientific problems.
Comments are closed.