Get ready for a deep dive into spatial biology and image analysis with Lorenz Rognoni, the Director of Image Data Science at Ultivue. Ultivue is a company specializing in spatial biology and Lorenz brings his wealth of knowledge in multiplex immunofluorescence (mIF) and image data science to this great conversation.
How To Overcome Challenges in Image Analysis for Spatial Biology w/ Lorenz Rognoni, Ultivue
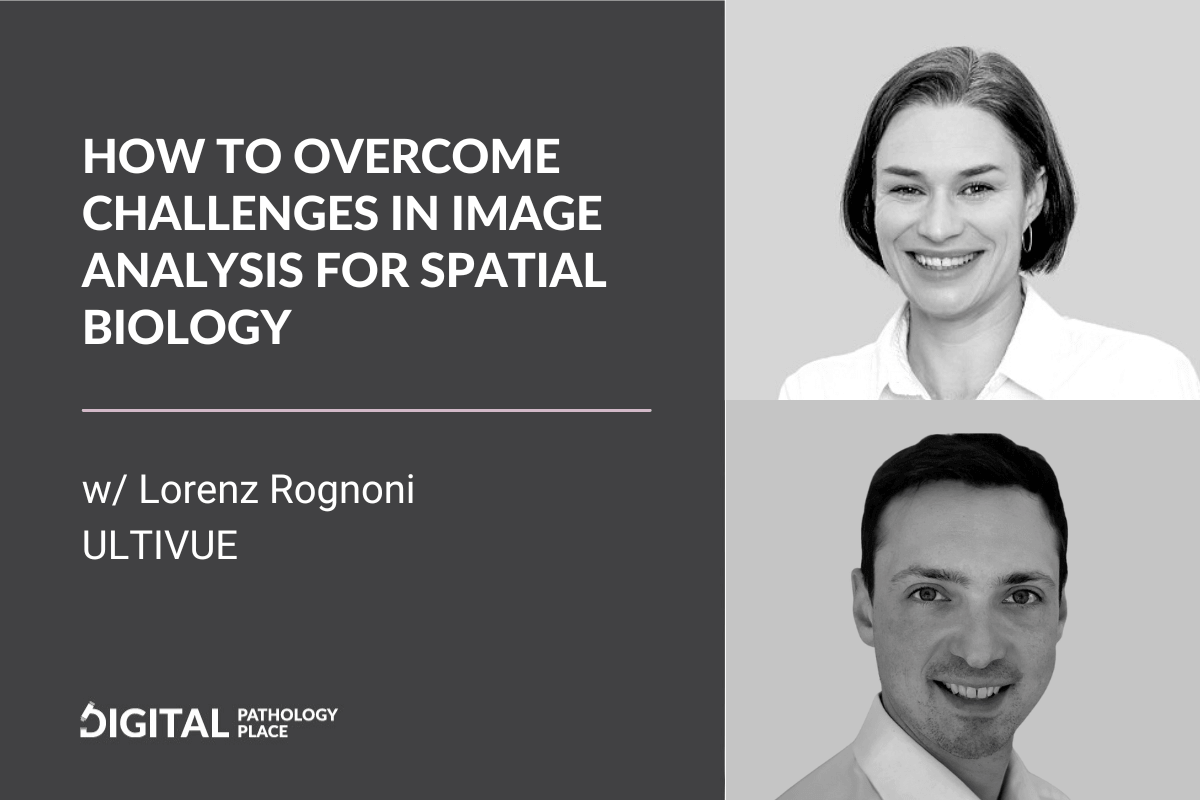
Exploring Spatial Biology and Image Analysis with Lorenz Rognoni
Multiplex IF: Challenges and Complexities
We kick off our discussion by addressing the inherent challenges in multiplex IF. The conversation spans a range of issues including tissue preparation artifacts, unique tissue morphology, and antibody-specific staining. The vast variability of tissues, differing across body regions, species, and health conditions, is a recurring theme. We also delve into the effectiveness of expert visual evaluation for traditional stains and the need for new strategies to interpret high-dimensional data.
Brightfield Imaging in Spatial Biology: Does it Still Play a Role?
Shifting gears, we discuss the role of brightfield imaging in spatial biology. Is there still space for brightfield if we want to learn the spatial interactions of cells in the tissue? Is this method not too limiting?
Lorenz underscores its continued relevance, particularly when robustness and scalability are prerequisites. He suggests transitioning to simpler methods like singleplex IF or even brightfield imaging, once research zeroes in on specific biomarkers of relevance with multiplex IF.
Transitioning from Image Analysis to Data Interpretation: Navigating the Pitfalls
Our conversation culminates in a look at the challenges and potential missteps in moving from image analysis to interpreting the data generated. Lorenz points out the crucial process of extracting meaningful insights from millions of cells, defining appropriate phenotypes, and considering the intricacies of downstream data mining.
Key Takeaways
- mIF is an exploratory method and the insights gained can later be transitioned ti simpler methods such as single market IF or IHC
- The spatial biology research relies on accurate cell segmentation and identifying the correct phenotypes of cells.
- Correct segmentation is the first step to explore the insights and this exploration is being done through informed data mining that takes into consideration all the information about the study. This is best done by an image data science team where image analysis scientists, data mining experts and pathologists work together.
Join us for this insightful conversation and gain a deeper understanding of the complexities and nuances of spatial biology and image data science with Lorenz Rognoni.
Get your “Digital Pathology Beginners Guide” E-book for free!Get it here.
Watch on YouTube
this episodes resources
- Lorenz Rognoni on LinkedIn
- Ultivue Official Website
Digital Pathology Place Resources
Transcript
Aleks: [00:00:00] Welcome everyone to the podcast. Today I’m gonna be talking to Lorenz Rognoni. He is the Director of Image Data Science at Ultivue, and Ultivue is a company specializing in spatial biology and image analysis and image data science is an integral component of spatial biology. In the classical biomarker work, you can still get away with visual evaluation, maybe one marker, two marker bright fields.
But when it comes to multiplex immunofluorescence, that’s no longer the case. So welcome, Lorenz to the podcast. How are you today?
Lorenz: Yeah, thanks. First of all, thanks for having me here, it’s really a pleasure to be here, your guest. And talk about this fantastic topic, special biology and how you can explore it with multiplex immuno fluorescence.
Yeah I’m brilliant. I’m very well.
Aleks: Fantastic. So today I wanna ask you just a few questions. First of all, what special challenges do multiplex. Immunofluorescent impose when it [00:01:00] comes to image analysis, my audience is more or less familiar with the brightfield image analysis, with analyzing IHC markers, singleplex, maybe double plex.
What challenges do we encounter do you encounter in your everyday work when it comes to multiplex IF?
Lorenz: Okay. Very good question. Also a broad one. Maybe let’s start.
Aleks: Yeah, you can narrow it down to the three most difficult challenges. I know that you can count them for an hour, but like when somebody’s starting doing multiplex IF, or let’s say they would wanna order multiplex IF images place to study it out of view, but maybe do their own image analysis. What do they need to think about?
Lorenz: Yeah. And let me start with a few words about how I got into this field, because I think it also explains what capabilities you need and then we can dive into the challenges and why you need these capabilities to actually tackle these challenges. From a training perspective, I’m a physicist and then went into biophysics, so taking the [00:02:00] methods.
Developed in physics to quantify biology and that’s what really drives me. How can you quantify biology? I spent a PhD in experimental biophysics, but then wanted to work closer to patient data, and that’s how I got into the field of digital pathology or computational pathology. But I wanted to have, let’s say the best possible tool to extract as much information as you can.
That’s what brought me to multiplex Immunofluorescence where you can really create a very high dimensional data set and yeah, then really assess a lot of information, which yeah. Is embedded in tissue. But you asked about the challenges and we are looking.
Aleks: Yes. Before we dive into that full disclosure, we used to work together at an image analysis company and we used to work together on multiplex IF projects, and now Lorenz is doing this full-time, so he’s an expert.
Lorenz: Yeah, that’s true. We yeah, we worked on the company specialized in image analysis and they are also on multiplex immunofluorescence. I also then spent quite some time doing [00:03:00] that for a big biopharma.
And one of my goals, or what really drives me is how can we make MIF and the analysis of MIF so robust that you can apply it on large scale in clinical trials and then really guide your decision making and then really increase the success rate on clinical trials. But yeah, back to the challenges, it’s done on tissue and therefore we also face the classical histology challenges being a tissue preparation artifact or like more mechanical artifact like folds, bubbles, or also tissue morphology like the process
of course, we have the whole chemistry like antibody, that can have un specific staining and so on. So that’s, I would say like the common basis, dependent of right field or like multiplex and high multiplex and I mean everyone who works in the field you as well know that tissue has an incredible heterogeneity.
So it can come from different parts of the body if you do translational work even from different species, you can have, different indications and this can create a huge [00:04:00] variability in tissue organization, morphologies or cellular morphologies, densely packed, very sparse.
And this can create a lot of difficulty if you want to described this with automated methods and I think that’s also the main reason that histology is, has still a very classic approach to look at the image as the highly trained expert tried to extract the embedded information, which I think still works very well on, let’s say the classic h e stain or as swiss had the bright field, the monoplex.
But as soon as you go to high dimensional data, you get this high dimensionality component on top, which means we add a lot of channels. If you use, if you start to look at yeah, dark images. So very often the expert is not trained on this kind of type of image. So you have also to learn this new way of looking at, you often have a very different dynamic range.
It’s way more linear that means you can have very light signal and very strong signal. You can have such a big bandwidth [00:05:00] that you can’t really look at it on a screen and you have to some scroll through the intensities. And again, this is something where computers, machines can really help, but of course you want to not have them do errors. You want to help your, let’s say, exploration.
Aleks: I remember because it’s like you say, you have to verify, you have to help. But when I was working on IF projects in contrast to brightfield, to confirm that the signal is coming from the right part of the tissue or to confirm the segmentation or anything that was done in the image analysis.
The exercise was multiplied by the number of markers. So for every markers I had to do it. And obviously by the third marker, you don’t remember how this image looked on the first marker. So it’s a good strategy to confirm to do qc, but it’s definitely not scalable for visual evaluation.
Lorenz: Yeah, that’s a good observation. So when you say we have one, I have image, and nowadays, depending on technology you can [00:06:00] also end up with 40 markers.
Aleks: Yeah you can make artwork with this like with all the rainbow colors you can have.
Lorenz: Yeah. Which means it’s not one image, it’s many images because also it’s a human. I think you can distinguish easily three colors maybe more, but then you create a new image, selecting the channels, depending on the cell type you want to explore. But then putting everything together is for human observers, incredibly difficult. So you have multiple dimensionality, let’s say, challenges, so one is the channel dimensionality, so you have more than your RGB channel or your normal color viewing that you’re trained on.
But you really have this multitude of information that you can selectively look at and do that very well, but Putting it together is really a challenge. So they are I think, having a good yeah. Imaging health strategy is mandatory. Of course, if you look at tissue, you can have like large resections with millions of cells, so you get also a lot of heterogeneity, as we said.
So you can get a lot of spatial, let’s say dimensionality on top and looking at all the cells on such a [00:07:00] huge area is also for a human very demanding task. So it’s easy to overlook, let’s say a rare say phenotype that maybe is the one you are actually interested in. So that’s another component which is difficult.
So again high dimension dimensionality is really for the human observer, big challenge, but not only for the human, also for the computer, because these images, they get extremely large. We are usually have images scanned with 12 or 16 bits or even 32. So it’s way larger per channel. Then you have multiple channels and maybe you have the large resection and the whole slide image. So you can end up with a hundred gigabyte of for one image.
Aleks: Is it a lot bigger than a bright field h and e like one if? How would you compare the size twice? Three times?
Lorenz: Yeah. So Brightfield, h and e you rarely go beyond the gigabyte. So you have three channels, but only an eight bit. You also often have better compression and so on, so they weigh smaller and all the software is optimized for the, these three channels. So that’s, the other part and yeah, what I wanted to say before is that you need [00:08:00] specialized data, specialized hardware for data storage, and then also for the computation.
And there nowadays, I mean you have two ways. I think the ideal way is really go into the cloud and then use scalable data and computation solution there. Or you are lucky and you’re working in a company with a huge data center and can do that locally. Yeah, with a high performance, compute cluster. But these are the two ways to approach really large data sets of MIF data. Doing that only locally on a computer. Yeah, it’s a little fun.
Aleks: Yeah. You’re gonna be spending a day working on one image maybe.
Lorenz: Yeah, I was talking about the marginality in the spatial, let’s say a component and they’re, millions of cells and that’s where really image analysis is key. So if you want to do spatial phenomics or spatial biology exploration, you need to get all the cells.
And all the phenotypes in that location. In theory, Like for an h and e, you could have a classification approach where you say responder versus non-responder. But if you want to go into this really high dimensional spatial biology, [00:09:00] you have to get all these cells and that’s where you need certainly computer-based image analysis.
And that’s also one of the challenges. So get accurate segmentation of either tissue regions. If you want to distinguish between two much stroma areas, for instance, or then down to the in individual cells. So getting really every single cell and then deciding for every cell in your panel, is it a positive cell or not.
And this is embedded in the usual, let’s say artifacts where you can have background coming from tissue preparation from, let’s say biological effects like autofluorescence causing red blood cells. So many different, let’s say additional challenges are there. And then of course, we can also spend some time talking about immunofluorescence signal and the variability that we often observe there.
Aleks: Question here, would you even now, after working so much in this space, is there even still a place for Brightfield if you’re serious about spatial biology or not [00:10:00] really? Or like in which, to which extent is there a space for Brightfield and when would you need to change to multiplex IF to have reliable and valuable data.
Lorenz: I think the theme is there. You need the right tool for your job and definitely like the high dimensionals spatial biology approach is very exploratory approach. So if you want to find, if we are now thinking of cancer, I dunno, in which indication are certain markers expressed or if you want to stratify patients or whatever.
So if you really want to explore mechanism of action and so on, so when you need the maximum information, then I think that’s a tool to go. And there are also multiple other omic techniques that then can also join in and you can combine them to really maximize your information there.
Once you have a clearer idea for instance, what type of biomarkers can help you stratify a patient population in a larger clinical trial? I think that’s a time where you then still should dial down, which could be still an IF, [00:11:00] having less markers, for instance. We like to reduce the complexity, especially than if you think of data mining afterwards.
The more parameters you have the more crowded your parameter spaces and the more complex the whole analysis becomes. So if you can make it simpler, you have also to deal with less noise. And then maybe it’s even the best because honestly they’re just more robust. If you can find an ifc or even an h and e morphology then can surrogate this signature for your patient certification, then you should do that in Brightfield.
Because just more robust if we think large clinical trial multicenter and the labs that they have there. It’s just the way to go and I think that’s key, so also having this transition then from the very high plex to the then very focused singleplex maybe.
Aleks: Okay. So what I’m hearing is it’s not really a competitive technique one to the other. It’s just, it has different use cases and when you are in the exploratory phase IF and the more markers you can explore, the higher [00:12:00] the chance of extracting meaningful information is. And once you have that, then you explore other methods to deploy it at scale, because as we said at the beginning IF is challenging.
And if you can reduce the how challenging the method is when you already have the insights, then of course you can deploy it in less stable conditions, which we. Know that clinical trial conditions are not always the most stable conditions, so you wanna have the most robust method.
Okay. Lorenz, you are the image data science director, right? So where are the greatest pitfalls on the transition from generating from image analysis to analyzing this data that might make this effort less meaningful? What are the problems that you can encounter? Something that can happen, like a mistake or something that can cause your downstream analysis to not provide value and how to mitigate this.
That’s the most important question. Where do we need to be aware of things that can go wrong?
Lorenz: We call [00:13:00] the team where we do the analysis, the image data science team, because it’s really, for many people the image analysis part is getting all the cells, but then it’s not stopping there. You then also have to decide which ones are the good cells, then define the biological relevant phenotypes.
And then make sense of this data because again, on the image we have millions of cells. But then depending on the size of your trial, you have way lower patient number, which means then the subsequent data mining is of a different dimensionality and then should also be treated differently. So the question is how can we extract from these millions of cells very good, like the best possible information?
And on one hand you want to boil it down, ideally to one number, let’s say, to have a certain score for a certain phenotype. But that often goes here, as we said, first, getting all the cells, then classifying them into positive, negative for every marker. And then again, defining the phenotype. So it means you, you multiply the data you have in addition of your millions of pixels that you already have before.
So it’s [00:14:00] really extracting in the end, the expert knowledge, so what’s a cell? What tissue regions do we have? What are the true phenotypes? So for instance, if we think of high dimensional data and co localization, so we can have the same marker staining, the same cell type and the same compartment even.
So like the membrane and then the question is, or the challenge is to really have a very accurate segmentation, but then also to really have a very accurate classification. So is this signal really coming from this cell or the adjacent cell? Because this can really change the whole view of the scene.
So do I have a double positive cell or is it two adjacent cells with different positivity? We try to apply the latest computer vision approaches, which in the end is deep learning or often also referred to as ai, to first get a very accurate segmentation, and then also with an independent deep learning model or classification approach, but then also classify the positivity for the different markers.
And that means for one dataset, let’s say we [00:15:00] often work with eight plexes. Because that’s like a sweet spot between dimensionality and channels, but then also how to handle and how quickly to analyze and there, we apply more than 10 deep learning models to do our full image analysis part, which then ends with a huge list or database of cells where every cell knows where it is, what neighbors it has, or which markers it was classified positive.
Then also some measurements like size and marker intensity and so on and then you get really a huge amount of data. And then next step is to make sense out of this data and to simplify. And that’s really, that depends a lot on the scientific question, how you move forward there and and we like to have a two step approach that we first to this.
Very standardized analysis, get all the cells, classify them and segment them as good as possible. Find the tumor stroma regions, for instance, if we work in cancer and then have a very standardized or where we then have this data. So we like to be as tool agnostic [00:16:00] as possible, and that’s maybe something we can sneak in.
So what this field really needs is like more common data format. Start with the image. But then of course it continues also with the image analysis data because depending on the tool you have, depending on the in-house custom software you wrote, you end up with a different format, which then means like community sharing of tools and having standards is really a problem.
And just having, for instance, one image standard would be already a huge leap forward. And we, for instance, tried to always to our projects, and one is the same open standard, the OMI standard but again it’s one approach. But reality is there’s a huge multitude of different standards and again, the same would also apply to the cell format.
So if we, similar to sequencing, would then converge to one standard to have this marginalized data output then also the following analysis, similar to the single cell sequencing analysis would be way more standardized and then also increase the whole community speed.
Aleks: I think super important and super [00:17:00] simple thing you said, making sense out of this data because having even the best perfect classification, segmentation of the image doesn’t guarantee that you’ll make sense of this data and you can still make a mistake and have the wrong conclusions even if your image analysis is perfect. So I think you guys have the advantage of having the two-step approach within one team and taking the scientific question into consideration so that you actually answered the question and your, the whole analysis is, tailored to answering this very question.
This probably influences how you design it and another thing that it is done in a standardized way. This cannot be emphasized enough. This is happening I think, across the whole field of digital pathology from. Images through annotation formats through the output formats. I think everybody, because we’re still, probably for us, it doesn’t feel like it’s a new field anymore because we spent already several years [00:18:00] working in this field.
But in the grand scheme of things, it’s always being compared to radiology. The radiology is more mature and we are still in the kind of freestyle phase. So every effort to make it less freestyle helps the new people. Coming and capitalizing on this knowledge and they don’t have to reinvent the wheel.
So I’m super happy to hear that you guys are definitely embracing that. Thanks so much for joining me today, and thanks so much for giving us the information and the things people need to be aware of when doing image analysis. My key takeaway image data science team is the way to go.
Because then you have both the image analysis, the pathology, and the data interpretation expertise in one place, those people can work together and give you the valuable insights that answer your question.
Lorenz: That’s correct. It’s really about having a multifunctional team where you have the different expertise because that’s what you need.
You need the computer science expert, you need the translational [00:19:00] expert, the pathologist, and like the tissue expert. Then also people that understand how are these, let’s say samples prepared and scanned. And so what are the different sources of artifacts? And they’re having, let’s say, an approach where you bring them all to one table, I think is key.
And yeah, we tried to do that and that’s how I encourage everyone who wants to work on this field, so really get all the different expertise at one table because you need them.
Aleks: Thank you so much. You have a great day!
Lorenz: Thank you. It was really a pleasure.
Aleks: Thank you so much for staying till the end. It means you are a real digital pathology trailblazer, so if you are doing any type of multiplex imaging, Or are simply interested in multiplex and digital pathology, I have something for you.
It’s the Digital Pathology Starter kit. Go ahead, click the link below and claim your starter kit. This is gonna give you a fantastic overview of what Digital Pathology is and how you can get involved right now, and I talk to you in the [00:20:00] next video.