This post is brought to you by Aiforia.
In tissue image analysis for pathology detecting structures of interest accurately is critically important. With classical, hand-crafted image analysis algorithms it was often a challenging task requiring extensive image analysis expertise. With deep learning-based image segmentation as part of the pathology toolbox, this task can now be solved by pathologists and scientists with no prior image analysis experience. One especially powerful tool is instance segmentation, offered by Aiforia‘s cloud-based artificial intelligence (AI) for image analysis platform.
IMAGE ANALYSIS FROM PATHOLOGIST’S POINT OF VIEW
When regarding tissue image analysis from the pathologist’s point of view, there are practically only two categories of structures of interest:
- regions
- and objects.
Regions are larger areas of the whole slide image (WSI) sharing similar characteristics, such as different types of tissues found on the same WSI or different tissue compartments within the same tissue such as tumor stroma and tumor epithelium.
Objects can refer to:
- subcellular structures
- e.g., cell nuclei
- fat vacuoles
- cellular processes
- cells
- e.g., inflammatory cells,
- epithelial cells
- neurons
- non-cell objects
- e.g., beta-amyloid plaques
- infectious agents
- and objects that are made out of cells, but where the cells themselves are not of interest
- e.g., renal glomeruli
- pancreatic islands
- nerve fibers.
Once the structures of interests are defined and identified, pathologists are interested in quantifying them in three different ways:
- They either just want to count them
- e.g., counting IHC positive cells such as the Ki67 positive cells in breast cancer,
- they might want to localize (delineate) and quantify their total area
- e.g., in the tumor stroma separation
- or fibrosis quantification
- or they might want to have it all: count, delineate and quantify the area of each object of interest.
For these pathology challenges three different computer vision solutions can be used:
- Object detection (where we just want to identify objects and count them)
- semantic segmentation (where we are interested in the total area of the class of interest, see below)
- and instance segmentation where we want to have it all.
WHAT IS IMAGE SEGMENTATION?
Image segmentation is the division of a digital image into meaningful segments (groups of pixels) that share similar characteristics. In the context of tissue image analysis, the meaningful segments share similar characteristics because they belong to the same category (so-called class) e.g., tumor stroma, tumor epithelium, inflammatory cells, kidney glomeruli.
The ability to automatically segment a tissue image is the foundation of the power and great utility of image analysis for pathology.
Correct image segmentation is the basis of quantitative image analysis.
Equipped with this tool, pathologists and scientists can quantify different pathological processes in the tissue. Segmentation can be used for delineating regions as well as objects which are later assigned to a particular class, based on their characteristics (computer vision task called “classification”). In deep learning-based image analysis the segmentation is coupled with classification in processes called:
- Semantic segmentation and
- Instance segmentation
In both processes the deep learning model is tasked with assigning each pixel in the image to a particular class, but instance segmentation uses an additional object detection step to separate all the individual instances belonging to the same class.
SEMANTIC SEGMENTATION VS INSTANCE SEGMENTATION
If we are now able to classify every pixel in the image with semantic segmentation does that not give us a tool for solving every image analysis problem in pathology? In many cases, such as region detection and area-based measurements yes, but…
What do we do if we are interested in separating individual objects belonging to the same class?
If the objects do not touch each other and are separated by pixels assigned to a different class, semantic segmentation is sufficient.
But what about cases where our objects of interests, e.g., cells or cell parts are touching? With semantic segmentation, they would be merged together and detected as a region and we are interested in the numbers and characteristics (e.g., size and shape) of the individual objects. To achieve that
we need to introduce an additional step of object detection. And this is exactly where instance segmentation comes in.
Not only does it enable us to classify every pixel in the image, but now we can also train the model to predict the individual objects belonging to the same class.
For example, when quantifying microglia in mouse brain tissue sections, both the number of microglia cells as well as the number and morphology of microglial processes is relevant for the researchers.
The microglia cells often touch each other, and several microglial processes can originate from the same stem in the cell body (soma). These touchpoints would cause merging of the objects (cells and their processes) if semantic segmentation were used.
In order to separate every microglial cell process branching from the soma we would need to use instance segmentation. This would give us the counts and the shapes of the microglial processes.
The morphological transitions of microglial processes have been shown to be crucial for microglial interactions with other cells. The detailed information about the length and shape of the processes could give us insights into understanding microglial functionality. If the researchers were able to characterize different categories of microglia based on the numbers and shapes of their processes, they could also correlate this information with other available data and gain even more granular insights.
Instance segmentation is the tool that can provide us with information about the shapes of our structures of interest as well as their counts. It can separate and delineate each microglial process, including processes that originate from the same stem.
To visualize how useful the instance segmentation is, let us take a step back and investigate different computer vision tasks and the granularity of the information they deliver:
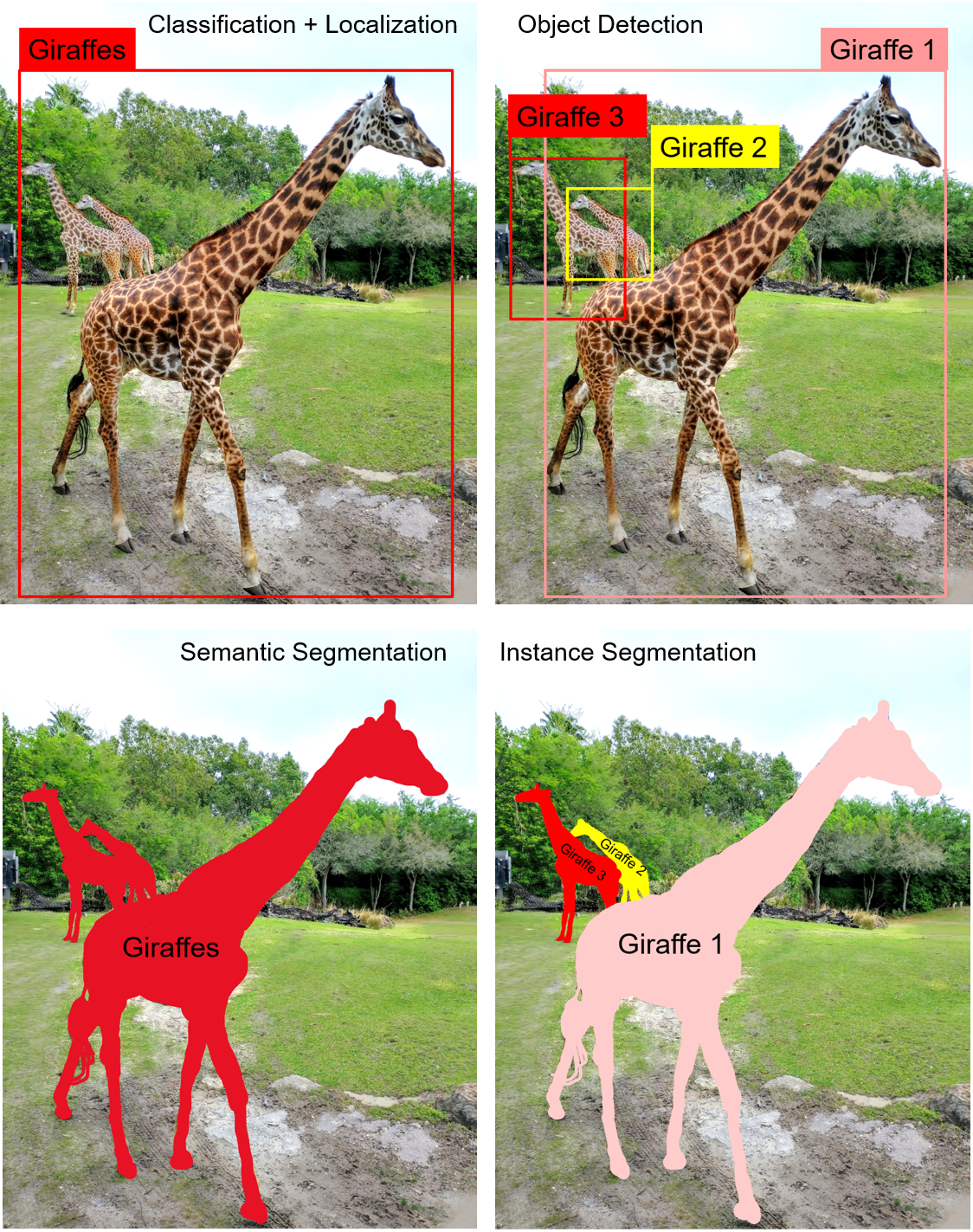
Instance segmentation is the combination of all other tasks – classification, localization, object detection and semantic segmentation. As such, this tool is very suitable for solving complex pathology problems such as in our example of microglial processes. This deep learning approach is extremely useful in pathology.
While many AI-based software solutions for tissue image analysis already provide the option of training a deep learning model to perform semantic segmentation, instance segmentation is not yet included.
Aiforia, a company specializing in AI for image analysis, recognized the importance of this powerful feature for solving pathology and medical imaging problems and incorporated instance segmentation into its software. Now it is at pathologists’ and scientists’ disposal integrated into a software platform for supervised deep learning model development. Depending on the problem to solve, users can choose to perform:
- semantic segmentation
- semantic segmentation with object detection (if they would just want to know the microglial counts)
- or instance segmentation (if they want to have detailed information, like the size and shape of each cell and their processes).
Each option is supported by intuitive annotation tools that let scientists start solving their image analysis problems immediately, without spending hours learning the software.
So, if you find yourself struggling with object delineation in your tissue image analysis project, evaluate if instance segmentation from Aiforia’s platform can help you. You can book a demo here.
Comments are closed.